Blog

Case Studies
How Gladia's multilingual audio-to-text API supercharges Carv's AI for recruiters
In today's professional landscape, the average workday of a recruiter is characterized by a perpetual cycle of administrative tasks, alternated by intake calls with hiring managers and interviews with candidates. And while recruiters enjoy connecting with hiring managers and candidates, there’s an almost universal disdain for the administrative side of the job.
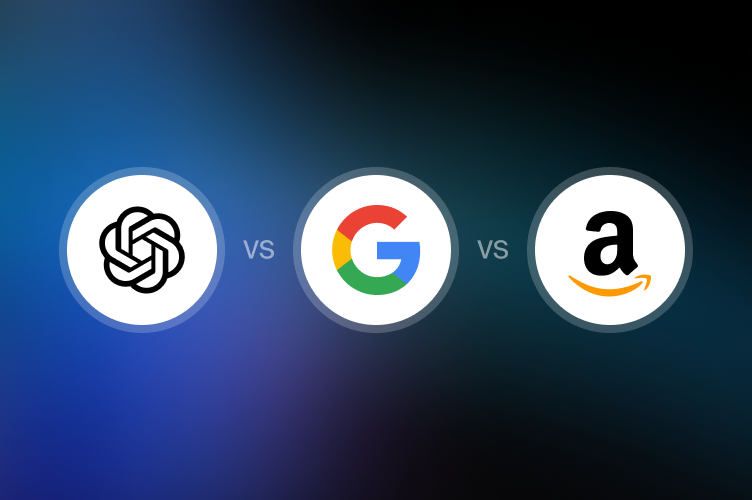
Speech-To-Text
OpenAI Whisper vs Google Speech-to-Text vs Amazon Transcribe: The ASR rundown
Speech recognition models and APIs are crucial in building apps for various industries, including healthcare, customer service, online meetings, and entertainment.
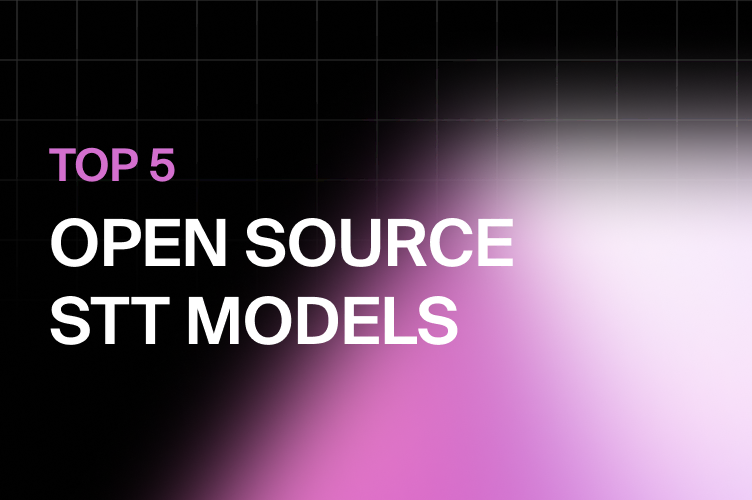
Speech-To-Text
Best open-source speech-to-text models
Automatic speech recognition, also known as speech-to-text (STT), has been around for some decades, but the advances of the last two decades in both hardware and software, especially for artificial intelligence, made the technology more robust and accessible than ever before.
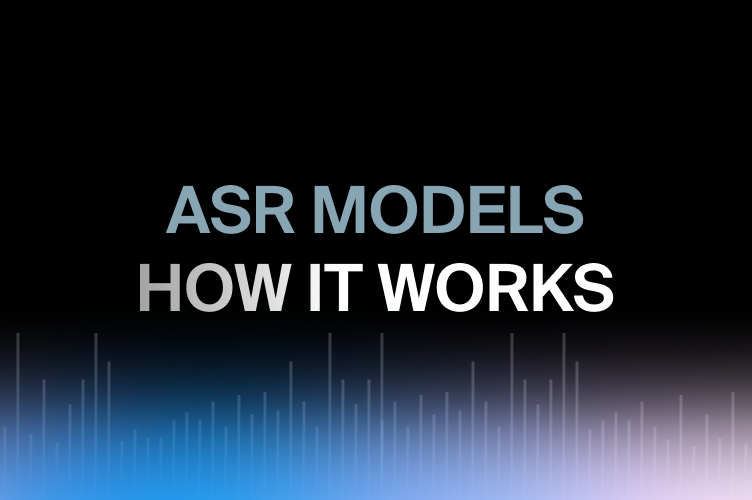
Speech-To-Text
How do speech recognition models work?
Automatic speech recognition (ASR) is a cornerstone of many business applications in domains ranging from call centers to smart device engineering. At their core, ASR models, also referred to as Speech-to-Text (STT), intelligently recognize human speech and convert it into a written format.
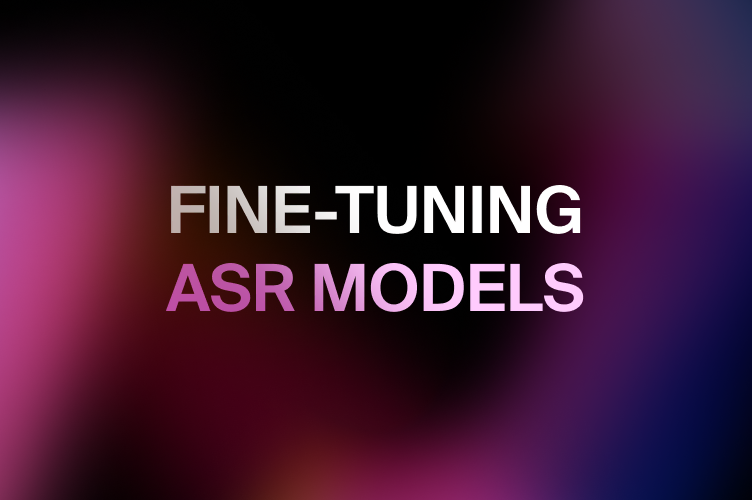
Speech-To-Text
Fine-tuning ASR models: Key definitions, mechanics, and use cases
Many modern AI models are built for general-purpose applications and require fine-tuning for domain-specific tasks. The fine-tuning process involves taking an existing model and training it further on domain-specific data. The additional training allows the model to understand the new data and improve its performance in a particular field.
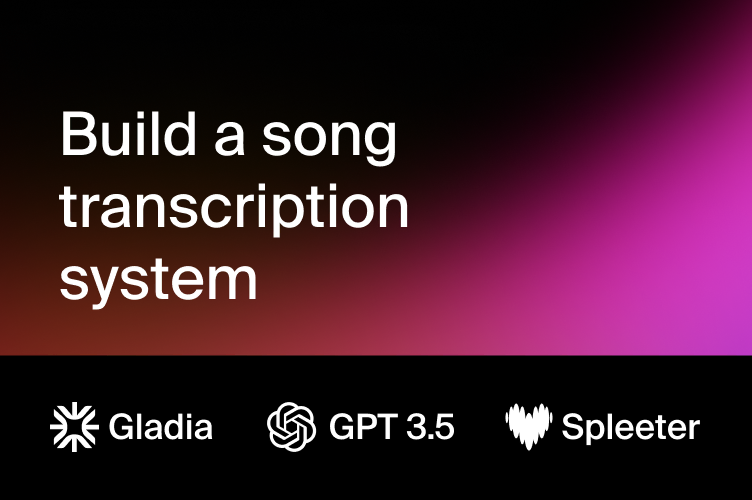
Tutorials
Building a song transcription system with profanity filter using Whisper, GPT 3.5 and Spleeter
The inception of music streaming gained initial popularity in 1999 with the founding of Napster, one of the pioneering streaming platforms. Millions of songs were available to listen to and download for free through the platform using the internet. One no longer needed to buy pre-recorded tapes, go to live shows, or tune into radio stations to listen to music.