How much does it really cost to host Whisper AI transcription?
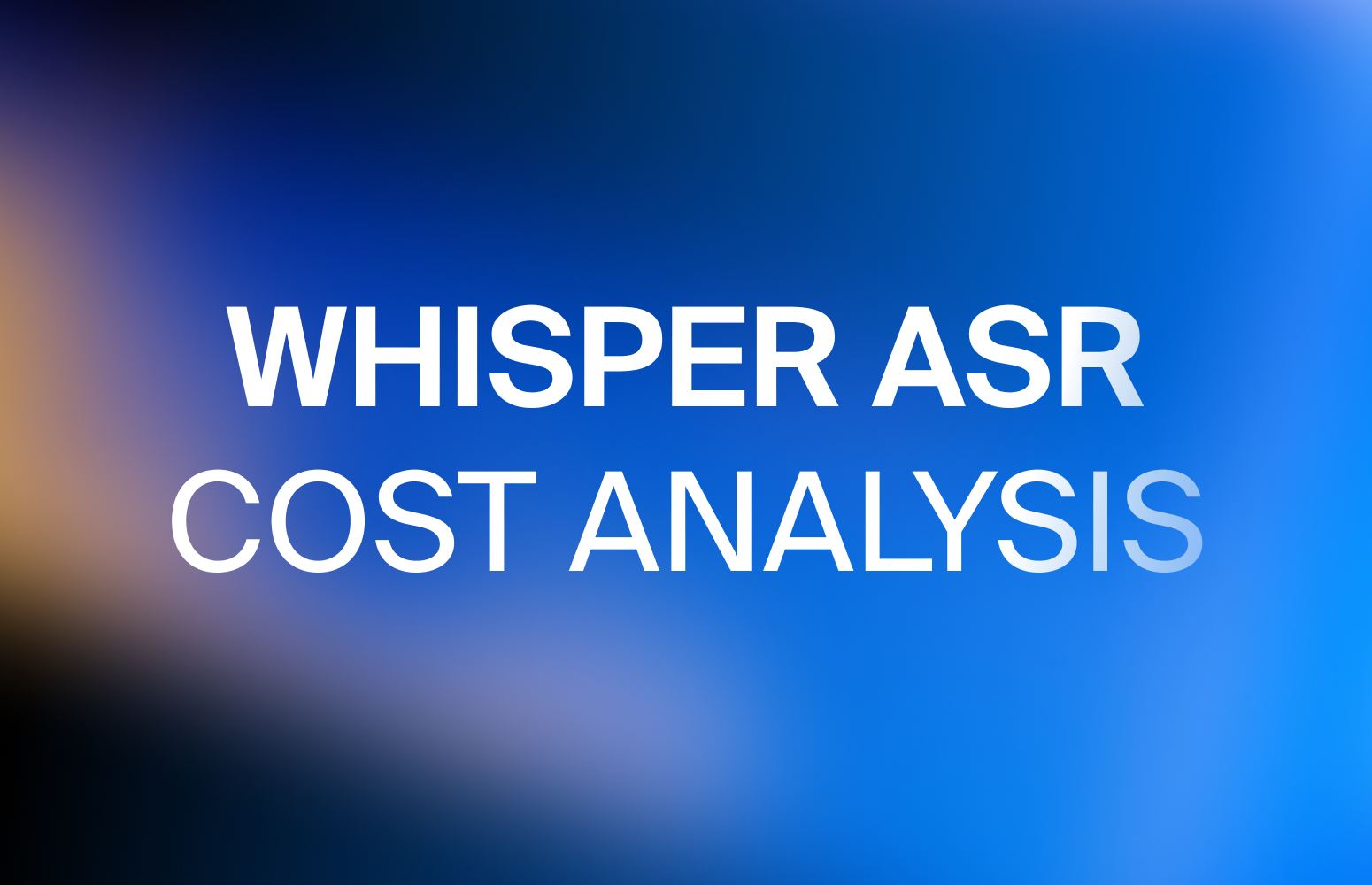
Open-source ASR models are often presented as the most cost-effective solution to embedding Language AI into your applications. But is that always the case? Here's our take.
What is Whisper AI transcription?
Open-source Whisper is a state-of-the-art automatic speech recognition (ASR) framework introduced by OpenAI in 2022. Trained on 680,000 hours of multi-language data, it became highly popular among indie developers and businesses alike for its accuracy and versatility in speech recognition – an excellent choice to power one’s apps with Speech AI.
Benefits for developers
1. Freedom of adaptation: Whisper ASR's open-source nature allows developers to modify and extend the system to meet their specific ASR project requirements, without being tied to predefined functionalities.
2. Variety of applications: Whisper enables developers to create an array of voice-enabled applications, such as transcription services, virtual assistants, voice-activated controls, and speech analytics, unlocking new possibilities for user interactions with technology.
3. Community collaboration: Developers building with Whisper benefit from the multiple DIY resources shared free of charge by the open-source community to advance and improve the functionalities they need for their products.
4. Cost-efficient solution: Utilizing an open-source ASR framework like Whisper can significantly reduce development costs, as it eliminates the need for expensive licensing fees associated with proprietary ASR tools.
The last point merits special attention: is it really always cheaper to host the open-source Whisper yourself than opt for an API? Let’s find out.
How much does it cost to host Whisper
While appearing cost-effective to acquire at the beginning, open-source models like Whisper often end up being more expensive when you take into account the total cost of ownership (TCO) required to host, optimize and maintain the ASR at scale.
There are a number of factors contributing to the TCO of speech-to-text technology:
Hosting
The cost of hosting text-to-speech technology begins at roughly 1$ per hour. That’s what it costs to run the CPU — which is responsible for processing the input text, applying natural language processing algorithms, and generating the speech output — and the GPUs, which are used to accelerate the natural language processing algorithms used to generate the speech output. While many other open source software, including Kaldi and Wav2Letter, can be used on CPU, Whisper in particular will require a fast GPU, especially for the most accurate, bigger version of the model.
Network
The cost of data transmission over the network is another significant factor in the TCO of speech-to-text technology. It varies based on the amount of data transmitted, the quality of the network connection, and your data plan. The higher the data transfer rates required by speech-to-text technology, the higher the network costs.
Authentication
Authentication is the process of verifying the identity of a user or device before allowing access to speech-to-text technology. Authentication costs can include the cost of hardware or software tokens, security certificates, and other authentication mechanisms.
Security
Security costs can include the cost of firewalls, antivirus software, intrusion detection and prevention systems, and other security measures. For companies operating in sensitive industries, such as healthcare or legal, security costs cannot be underestimated.
Developers
Here, we arrive at the main cost — your human capital.
Whisper was never designed to be production-ready, and has inherent limitations (e.g. hallucinations, limited functionalities) that require substantial engineering adjustments in order to function at scale. To built on top of it effectively and fine-tune it sufficiently to your specific use cases, you’ll most likely need at least two developers. More often than not, other specialists, such as data scientists and project managers, are also needed. You’ll need to invest time and money into finding them: keep in mind that AI/ML experts are still a dime a dozen in today’s talent market.
Once you’ve recruited the right senior software developers, you’ll need to pay them yearly salaries of at least $88,000 if US-based and $50,000 if in Europe. Taking a typical team 'two-pizza team' of 5-6 people, this translates to about $500,000 per year of labor costs — which is a very significant number.
Supervision and maintenance
The complexity of the speech-to-text technology means that additional support is often required, including software updates, patches, bug fixes, and technical support. That’s why you need to reserve an additional 20% on top of your staffing budget simply for maintenance and support costs. Like with any other open source solution, you need to be ready to assume all downtime and maintenance risk.
Certification
Last but not least, companies operating in industries with strict compliance standards may want to get their speech-to-text solutions officially certified. Needless to say, the rigorous testing and evaluation involved in this process, as well as security and maintenance costs, will further add to the TCO of speech-to-text technology.
To summarise, because of the operational cost of integrating a new solution into your existing workflows and then supporting a dedicated team of specialized staff, the TCO of a speech-to-text solution easily amounts to an annual budget from $300k- $2 million.
Whether this price tag is worth it will depend largely on your use case and project needs. For many companies, opting for another ASR model or a pre-packaged API would make more sense. More on this topic in our dedicated blog post.
About Gladia
At Gladia, we built an optimized version of Whisper in the form of an API, adapted to real-life enterprise use cases and distinguished by exceptional accuracy, speed, extended multilingual capabilities and state-of-the-art features, including speaker diarization and word-level timestamps.

Contact us
Read more

Speech-To-Text
Enhancing CX with AI: Key Trends to Watch 2024
AI is transforming contact centers at an accelerating pace. Speech AI technologies are at the forefront of this revolution, enabling companies to provide better customer experiences through a combination of advanced agent-assist techniques and fully automated interactions that feel natural and human-like.
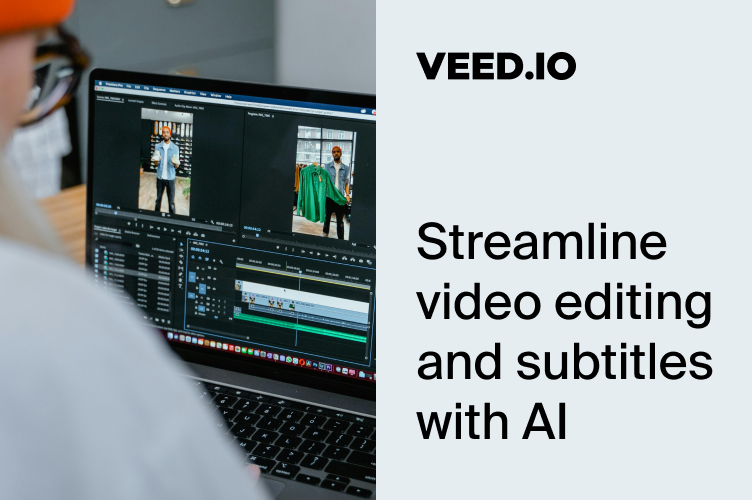
Case Studies
How VEED is streamlining video editing and subtitles with AI transcription
User-generated content has become a cornerstone of the internet-driven economy. As part of this shift, various platforms have emerged to provide easy-to-use tools to create high-quality video content in a matter of minutes — with AI transcription playing a foundational role in their product development.

Tutorials
How to build a speaker identification system for recorded online meetings
Virtual meeting recordings are becoming increasingly used as a source of valuable business knowledge. However, given the large amount of audio data produced in meetings by companies, getting the full value out of recorded meetings can be tricky.